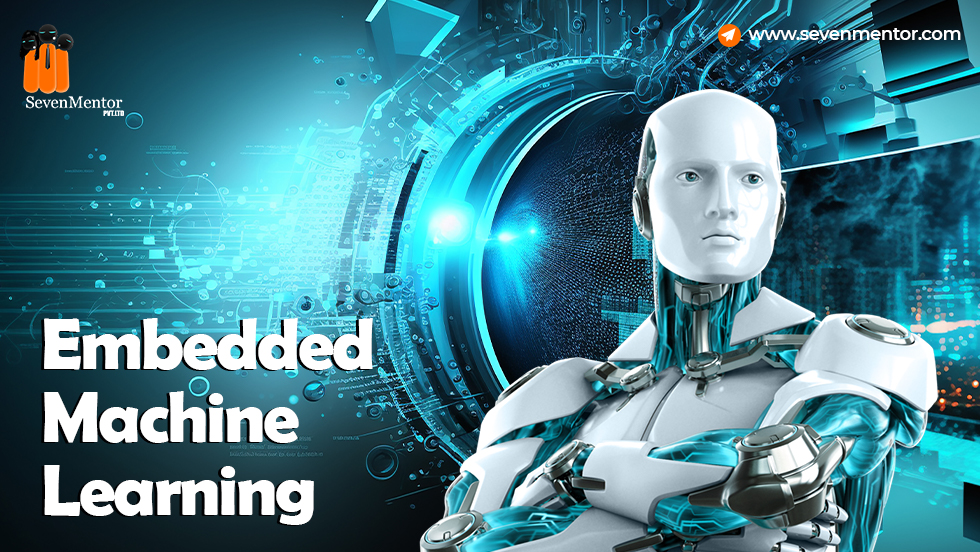
A machine learning paradigm that sits in between supervised and unsupervised learning is called semi-supervised learning. In semi-directed learning, the dataset contains a combination of named and unlabeled data of interest. The objective is to use the two sorts of information to fabricate prescient models or concentrate helpful data.
Here is an outline of semi-directed learning:
Named Information:
Marked information comprises of info tests matched with relating yield names or target values. These marked information focuses are utilized to prepare managed learning models, where the calculation figures out how to make expectations in light of info yield matches.
Unlabeled Information:
Unlabeled information comprises of info tests without relating yield names. In contrast to regulated realizing, where each datum point is named, unlabeled information is bountiful and frequently simpler to secure in genuine situations.
Model Preparation:
In semi-administered learning, the calculation uses both named and unlabeled information during the preparation cycle. The marked information is utilized likewise to administered realizing, where the calculation gains from named guides to make forecasts or concentrate designs.
Additionally, by incorporating information from the unlabeled samples into the learning process, the unlabeled data are utilized to enhance the model’s performance. This can assist the model with learning a superior portrayal of the basic information conveyance and sum up more successfully to concealed information.
Pseudo-labeling:
Pseudo-labeling, in semi-supervised learning, is a common strategy in which the model predicts on unlabeled data and gives these predictions pseudo-labels. The supervised model is trained by combining the labeled and pseudo-labeled data.
Pseudo-marking can assist the model with using the data contained in the unlabeled information to work on its exhibition, particularly in situations where named information is scant or costly to get.
Co-training:
One more methodology in semi-managed learning is co-preparing, where various models are prepared on various subsets of elements or perspectives on the information. Each model is trained using a mix of labeled and unlabeled data, and during the training process, they share information to boost each other’s performance.
Co-preparing is especially helpful when the dataset contains various modalities or wellsprings of data that can be utilized to upgrade the growing experience.
Semi-regulated learning is particularly helpful in situations where marked information is restricted or exorbitant to gain, however unlabeled information is promptly accessible. By actually using both marked and unlabeled information, semi-directed learning can work on model execution, upgrade speculation, and lessen the requirement for a lot of named information. It has applications in different spaces, including regular language handling, PC vision, and discourse acknowledgment.
Visit : Machine Learning Training in Pune | Machine Learning Course in Pune