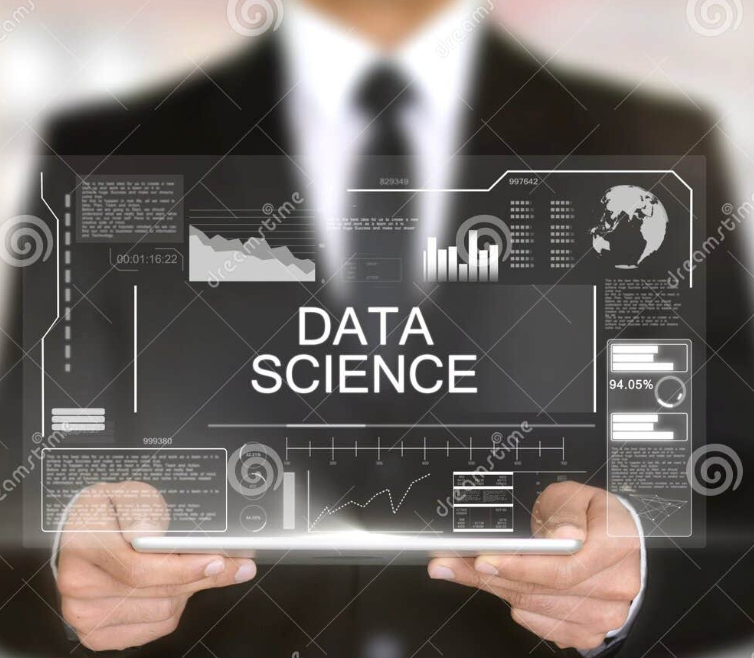
The hardest part of being a data scientist can vary depending on individual strengths, preferences, and the specific context of the work. However, several common challenges are often cited by data scientists:
Visit For More Info – Data Science Classes in Nagpur
-
Data Preparation and Cleaning: Preparing and cleaning data is often cited as one of the most time-consuming and challenging aspects of data science. Dealing with missing values, outliers, inconsistencies, and noise in the data requires careful attention to detail and domain expertise.
-
Problem Formulation: Defining the problem and framing it in a way that is both feasible and impactful can be challenging. Identifying the right questions to ask, understanding the business context, and aligning stakeholder expectations are essential for successful problem formulation.
-
Feature Engineering: Selecting and engineering relevant features from raw data to improve model performance can be complex and requires domain knowledge, creativity, and experimentation. Finding the right balance between simplicity and complexity in feature engineering is often a delicate task.
-
Model Selection and Tuning: Choosing the right machine learning algorithms and hyperparameters for a given problem, and tuning them for optimal performance, can be challenging. It requires a deep understanding of the underlying algorithms, as well as experience in experimentation and evaluation.
- Visit For More Info – Data Science Course in Nagpur
-
Interpretability and Explainability: Building models that are not only accurate but also interpretable and explainable is crucial, particularly in domains where transparency and accountability are important. Balancing model complexity with interpretability can be a challenging trade-off.
-
Deployment and Integration: Moving from prototyping to production involves deploying models into real-world systems and integrating them with existing infrastructure. This process can be complex and requires collaboration with software engineers, DevOps teams, and other stakeholders.
-
Communication and Stakeholder Management: Effectively communicating complex technical concepts and findings to non-technical stakeholders can be challenging. Data scientists need to be able to translate their insights into actionable recommendations that resonate with decision-makers.
-
Keeping Up with Advancements: Data science is a rapidly evolving field with new techniques, algorithms, and tools emerging regularly. Staying updated on the latest advancements and continuously learning new skills can be challenging but essential for professional growth and relevance.
Overall, being a data scientist requires a combination of technical expertise, problem-solving skills, domain knowledge, and effective communication abilities. While each aspect of the role presents its own challenges, overcoming them can lead to rewarding outcomes and impactful contributions in the field of data science.
Visit For More Info – Data Science Training in Nagpur